Ayurveda and Siddha systems polyherbal formulations to treat COVID-19 caused by SARS-CoV-2 and brief insight on application of Molecular Docking and SWISS Target prediction tools to study efficacy of active molecules
Keywords:
COVID-19, SARS-CoV-2, Kabasura churan, Maha Sudarshan churan, Siddha system, Ayurvedic system of medicineAbstract
Ayurveda and Siddha systems are the two ancient medical systems originated in India more than 4000 years ago had given many formulary and treatment methods against influenza like infections. Kabasura churan from Siddha system and Maha sudharshan churan from the Ayurvedic system are the two major formulations along with many other individual herbs mentioned in the texts to treat Influenza like infections. Kabasura churan and Maha Sudarshan churan both have antipyretic, analgesic and anti-inflammatory effects. Both formulations were prepared according to Siddha and Ayurvedic texts. Herbs mentioned in both formulations like Turmeric, Tulsi (Basil), Kalmegh (Andrographis), Black Pepper, Liquorice (Mulethi), and Dronapushpi (Leucas) etc., had direct antiviral effect. Herbs like Aswagandha, Ginger, Guduchi (Tinospora), Kulanjan (Galangal) etc., had immunomodulatory and anti-inflammatory effect. Active compounds from different herbs were selected to study their antiviral activity through molecular docking algorithm. Application of modern of tools like Bioinformatics and Highthroughput screening methods can predict the efficacy of the ancient documented formulations and can be compared as per their literature. Compounds like curcumin, Glycyrrhizin, Ursolic acid, Quercetin, Andrographolide, Coumarins etc. were showed polyspecific activity like inhibition of Spike protein, Furin, Main Protease (Mpro) and Papain like Proteases (PLpro). Thus we propose use of Kabasura churan and Maha Sudharshan churan as alternative complementary medicine as a palliative treatment against COVID-19 caused by SARS-CoV-2 by conducting proper Randomized Clinical Trials
References
Anonymous. The Ayurvedic Pharmacopoeia of India. Part II, Vol- I, Appendix 2.2.8. Govt. of India, Ministry of Health and Family Welfare, Department of Ayurveda, Yoga & Naturopathy, Unani, Siddha and Homeopathy (AYUSH). 1st Edition. New Delhi: The Controller of Publication; 2006. p.141 2. Anonymous. The Ayurvedic Pharmacopoeia of India. Part II, Vol- I, Appendix 2.2.8. Govt. of India, Ministry of Health and Family Welfare, Department of Ayurveda, Yoga & Naturopathy, Unani, Siddha and Homeopathy (AYUSH). 1st Edition. New Delhi: The Controller of Publication; 2006. p. 159 3. Byrne R., Schneider G.. In silico target prediction for small molecules. Methods Mol. Biol. 2019; 1888:273–309 4. Bikadi, Z., Hazai, E.Application of the PM6 semi-empirical method to modeling proteins enhances docking accuracy of AutoDock J. Cheminf. 1, 15 (2009) 5. Chauhan S, Pundir V, Sharma A. Pharmacopeial standardization of mahasudarshan churna: a polyherbal formulation. Journal of Medicinal Plants Studies 2013;1(2):13-18 6. Ding H., Takigawa I., Mamitsuka H., Zhu S.Similarity-based machine learning methods for predicting drug-target interactions: a brief review. Brief. Bioinformatics. 2014; 15:734–747 7. F. J. Solis and R. J. B. Wets Minimization by Random Search Techniques Mathematics of Operations Research 6 (1), 19-30 (1981) 8. Gunapadam Mooligai Vaguppu. Chennai: Siddha Medical Council, Tamilnadu K S Murugesamudalier,4-4, 1988 9. Gfeller D., Grosdidier A., Wirth M., Daina A., Michielin O., Zoete V. SwissTargetPrediction: a web server for target prediction of bioactive small molecules. Nucleic Acids Res. 2014; 42:W32–W38. 10. G. M. Morris, D. S. Goodsell, et al. Automated docking using a Lamarckian genetic algorithm and an empirical binding free energy function Journal of Computational Chemistry 19 (14), 1639-1662 (1998) 11. J. F. Blake, “Chemoinformatics-predicting the physicochemical properties of “drug-like” molecules,” Current Opinion in Biotechnology, vol. 11, no. 1, pp. 104–107, 2000 12. Literature Review on Siddha Herbal Formulations (Kudineer) Available for The Management of Dengue Harihara Mahadevan, Vanmathi Palraj, International Journal of Pharmacology and Clinical Sciences, 5: 90-96, 2016 13. LavekarGS, Ravishankar B, Rao SV, Shukla VJ, Ashok B K, Gaidhani SN. Safety study of a selected ayurvedic formulation: Mahasudarshan Ghanvati. Indian Drugs 2009; 46(11):20-29 14. Mitchell, J. B. O. Machine learning methods in chemoinformatics. WIREs Comput. Mol. Sci. 4, 468–481 (2014). 15. Siddha Maruthuvam, Chennai: Siddha Medical Wing, Department of Indian Medicine and Homeopathy K N Kuppusamymudaliyar, Government of Tamilnadu: 1987 16. Tian, S. et al. The application of in silico drug-likeness predictions in pharmaceutical research. Adv Drug Deliv Rev 86, 2–10 (2015). 17. T. A. Halgren Merck molecular force field. I. Basis, form, scope, parametrization, and performance of MMFF94 Journal of Computational Chemistry 17 (5-6), 490-519 (1998) 18. Pires, D. E. V., Blundell, T. L. & Ascher, D. B. pk CSM: Predicting Small-Molecule Pharmacokinetic and Toxicity Properties Using Graph-Based Signatures. J. Med. Chem. 58, 4066–4072 (2015). 19. Zoete, V., Daina, A., Bovigny, C. & Michielin, O. Swiss Similarity: A Web Tool for Low to Ultra High Throughput Ligand-Based Virtual Screening. J. Chem. Inf. Model. 56, 1399–1404 (2016). 20. https://www.rcsb.org/ 21. https://www.ncbi.nlm.nih.gov/books/NBK554776/figure/article-52171.image.f3/ 22. Manikyam HK & Joshi SK (2020) Whole Genome Analysis and Targeted Drug Discovery Using Computational Methods and High Throughput Screening Tools for Emerged Novel Coronavirus (2019-nCoV). J Pharm Drug Res, 3(2): 341-361. 23. Hemanth kumar Manikyam & Joshi Sunil K (2020) Nicotinamide, Folic Acid and Derivatives as Potent Inhibitors of Inflammatory Factors against Novel Corona Virus Infection, Acta Scientific Pharmacology (ASPC) Volume 1 Issue 5 May 2020
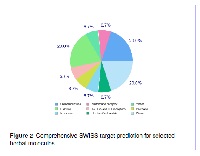